What is Data Science?
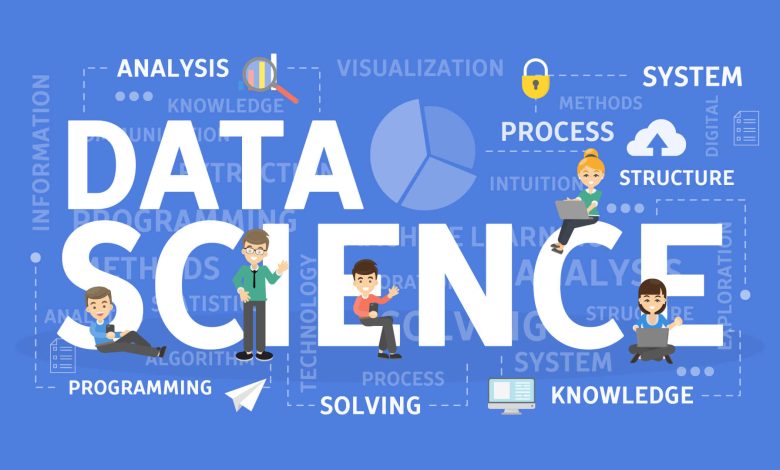
What is Data Science?
Data Science is an interdisciplinary field that uses scientific methods, algorithms, and systems to extract insights and knowledge from structured and unstructured data. It combines principles from statistics, mathematics, computer science, and domain expertise to analyze and interpret complex data sets. In today’s data-driven world, data science plays a crucial role in decision-making processes across various industries.
This article explores the core components of data science, its applications, benefits, challenges, and future trends.
Understanding Data Science
Data science encompasses several key components:
- Data Collection – The first step in data science is gathering data from various sources. This can include databases, APIs, web scraping, and direct user inputs. The quality and quantity of the data collected significantly impact the analysis.
- Data Cleaning and Preparation – Once data is collected, it often requires cleaning and preprocessing. This step involves removing duplicates, handling missing values, and transforming data into a suitable format for analysis. Proper data preparation is essential for accurate results.
- Data Analysis – In this phase, data scientists use statistical methods and algorithms to analyze the data. This analysis can involve exploratory data analysis (EDA), which helps identify patterns, trends, and anomalies within the data.
- Machine Learning – Data science frequently incorporates machine learning techniques. These algorithms enable systems to learn from data and make predictions or classifications based on new input. Common machine learning methods include regression, clustering, and neural networks.
- Data Visualization – Communicating findings effectively is crucial in data science. Data visualization tools, such as charts, graphs, and dashboards, help present complex data in a clear and understandable manner, making it easier for stakeholders to grasp insights.
- Deployment and Monitoring – Once a data model is built, it needs to be deployed into a production environment. Continuous monitoring ensures that the model performs well over time and remains accurate as new data is introduced.
Applications
Data science has a wide range of applications across various industries. Here are some notable examples:
- Healthcare – In healthcare, data science plays a vital role in predictive analytics, patient care optimization, and personalized medicine. By analyzing patient data, healthcare providers can identify trends, improve treatment outcomes, and enhance operational efficiency.
- Finance – Financial institutions use data science for risk assessment, fraud detection, and investment analysis. By analyzing historical data and market trends, data scientists help firms make informed decisions and mitigate potential risks.
- Retail – Retailers leverage data science to enhance customer experiences and optimize inventory management. By analyzing purchasing patterns, businesses can personalize marketing strategies, forecast demand, and improve supply chain operations.
- Marketing – In marketing, data science enables targeted advertising and campaign optimization. Analyzing consumer behavior and preferences allows marketers to create tailored strategies that drive engagement and conversions.
- Transportation – Data science is integral to optimizing logistics and transportation networks. Companies like Uber and Lyft use data analytics to match riders with drivers, predict demand, and optimize routes, enhancing overall efficiency.
- Sports – In the sports industry, data science helps teams analyze player performance, develop strategies, and enhance fan engagement. By leveraging data, teams can make data-driven decisions to improve outcomes on and off the field.
Benefits of Data Science
The adoption of it offers several advantages:
- Informed Decision-Making – It enables organizations to make decisions based on data rather than intuition. This data-driven approach leads to better outcomes and minimizes risks.
- Improved Efficiency – By automating data analysis and decision-making processes, it increases operational efficiency. Organizations can identify areas for improvement and streamline processes effectively.
- Competitive Advantage – Companies that leverage it can gain a competitive edge by understanding market trends and consumer behavior. This knowledge allows them to innovate and adapt to changing conditions.
- Enhanced Customer Experiences – Data science helps organizations better understand their customers. By analyzing preferences and behaviors, businesses can create personalized experiences that drive satisfaction and loyalty.
- Cost Reduction – By optimizing operations and improving resource allocation, data science can lead to significant cost savings. Organizations can identify inefficiencies and implement data-driven strategies to reduce expenses.
Challenges of Data Science
Despite its many benefits, it also faces several challenges:
- Data Quality – The effectiveness of data science relies on the quality of the data collected. Inaccurate, incomplete, or biased data can lead to flawed analysis and misleading conclusions.
- Skill Shortage – The demand for skilled data scientists continues to grow, but there is a shortage of qualified professionals in the field. This skill gap can hinder organizations’ ability to harness data science effectively.
- Data Privacy and Security – With the increasing amount of data collected, concerns about privacy and security are paramount. Organizations must implement robust measures to protect sensitive information and comply with regulations.
- Integration of Data Sources – Many organizations struggle with integrating data from various sources, which can lead to fragmented insights. Establishing a unified data infrastructure is crucial for comprehensive analysis.
- Overfitting and Underfitting – In machine learning, overfitting occurs when a model is too complex and learns noise instead of the underlying pattern. Underfitting happens when a model is too simple to capture the data’s complexity. Balancing these issues is critical for accurate predictions.
The Future of Data Science
The future of data science is promising, with several trends shaping its development:
- Automation of Data Science – Tools that automate data preparation, analysis, and model building are becoming more prevalent. This automation will make it more accessible to non-experts and streamline workflows for data professionals.
- AI and Machine Learning Integration – As AI technologies continue to evolve, they will become more integrated into data science practices. This integration will enhance predictive analytics and enable more advanced data-driven solutions.
- Increased Focus on Ethics – As data science plays a larger role in decision-making, ethical considerations will gain prominence. Organizations will need to prioritize ethical data use and address biases in algorithms.
- Real-Time Analytics – The demand for real-time data analysis is increasing across industries. As technology advances, organizations will be able to make decisions based on live data, improving responsiveness and agility.
- Enhanced Collaboration – Cross-disciplinary collaboration between data scientists, domain experts, and business leaders will become more critical. This collaboration will ensure that data-driven insights align with organizational goals.
Conclusion
Data Science is a vital field that enables organizations to harness the power of data for informed decision-making. By combining statistical analysis, machine learning, and domain expertise, data science provides valuable insights that drive innovation and improve outcomes across various industries.
As technology continues to evolve, data science will play an even more significant role in shaping the future of businesses and society. Embracing data-driven practices will empower organizations to navigate an increasingly complex and data-rich landscape.